Invited Speaker---Dr. Suely Oliveira, Professor
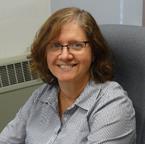
Department of Computer Science, The University of Iowa, USA
Biography: Suely Oliveira is a Professor in Computer Science at the University of Iowa, with a joint appointment in Mathematics, member of the Applied Mathematical and Computational Sciences and Informatics Programs. She received her PhD from the University of Colorado in 1993, followed by a postdoctoral fellowship at the Australian National University (1993-1994), Assistant Professor in Computer Science at Texas A&M University (1994-1998). She was a visiting Researcher at Universidade de Sao Paulo (2009-2010).
Her research is on the development of new algorithms and software for new methods in Computer Science and Engineering. Earlier she developed multilevel clustering algorithms for protein-protein networks, and new optimization methods for matrix based on clustering. From clustering she moved to other machine learning topics such as classification and neural networks. Particularly, she addresses problems with large amounts of data where new optimization algorithms or parallel computing is essential. She teaches courses in the area of parallel computing, algorithms and discrete mathematics for CS students.
Dr. Oliveira has published over 50 articles, chaired over 10 PhD Committees and is co-author of the books "Writing Scientific Software", Cambridge University Press and "Building Proofs: A Practical Guide", World Scientific.
Speech Title: Variational Autoencoders for Baseball Player Evaluation
Abstract: In the sporting world, baseball has been quicker to embrace the use of data analytics than any other sport, as detailed baseball statistics have become readily available in large and diverse quantities to the general public. Professional baseball teams use this data to develop game plans and evaluate players. In this work, we explore the latter by using a Variational Autoencoder (VAE), a special class of artificial neural networks. Specifically, we wish to relate a player’s season-long batting statistics with the latent skills that a professional athlete needs to succeed in the MLB. In the growing field of sports analytics, we find this work incredibly important as it provides a novel, flexible, and powerful method to predict specific athletic skills based on years of recorded statistics.