Invited Speaker---Dr. Dexian Huang, Professor
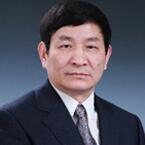
Department of Automation, Tsinghua University, China
Biography: Dexian Huang is a professor of the Department of Automation, Tsinghua University, Beijing, China. He received the B.S. degree in chemical automation and instrument from the China University of Petroleum (Huadong), Shangdong Province, China, the M.S. degree in industrial automation from the China University of Petroleum (Beijing), Beijing, China, and the Ph.D. degree in control theory and control engineering from Tsinghua University, Beijing, China, respectively. From 1992 to 1995, he was an Associate Professor with the Department of Automation, China University of Petroleum (Huadong). From 1995 to 2000, he was a Professor with the Department of Automation, China University of Petroleum (Huadong). Since 2000, he has always been a Professor with the Department of Automation, Tsinghua University. His research interest includes the modeling, simulation, control and real-time optimization of process industry. He served as Deputy Director of the Process Control Committee and Member of Chinese Association of Automation, served as Vice-Director of Information Technology Committee of Chemical Industry and Engineering Society of China, etc. He served as editorial committee member of Chinese Journal of Chemical Engineering Chinese Journal of Chemical Engineering, etc. He has published several books, “Process Control System”, “Chemical Process Advanced Control”, etc. He has published more than 200 articles in the " IEEE Transactions", "Automatica", "Control Engineering Practice" , "Journal of Process Control”, “Expert Systems with Applications”,”AIChE Journal", "Computers & Chemical Engineering", “Computers and Operations Research”, “American Control Conference (ACC)”, “International Conference on Systems, Man, and Cybernetics (SMC)”, etc. He won a second-class national prize for scientific and technological progress and more than a dozen provincial and ministerial Awards.
Speech Title: Recent Advances on Industrial Process Data Analytics Induced by Slowness Principle
Abstract: Safe and efficient operations of complex industrial processes are of significant importance to maximize economic and social benefits. Data-driven methodologies and machine learning techniques have shown great promises in modelling complicated industrial processes, and thus have been widely applied to areas such as process monitoring, fault diagnosis, and quality prediction. However, traditional data-driven models fall short of making full use of process data. In particular, their physical meanings are far from interpretable, thereby leaving a large quantity of information unused. This leads to a series of practical limitations, such as high false alarm rates and low-quality prediction accuracies, thereby increasing economic costs and potential safety risks in the process industry. To address these issues, the design of data-driven models must be intimately integrated with dynamic characteristics of industrial process data.
In this talk, we introduce a tailored process data analytics framework arising from slowness principle, which well exemplifies the spirit of representation learning and shapes a systematic data-driven paradigm. Its exclusive attractiveness lies in that distributions of both steady state and dynamic behaviours of process data can be simultaneously delineated, thereby leading to a comprehensive interpretation of process operation status, as well as successful applications in process monitoring, fault diagnosis, control performance assessment and quality predictions. Special attentions will be placed on a holistic design of intelligent alarm systems for process industries. The developed methodology enables effective discriminations between nominal operating condition deviations and process dynamics anomalies. It helps deciding whether the process model should be updated in an automatic way, and hence reduces manual labor and ensures the long running of industrial processes.