Invited Speaker---Dr. Maurizio Marchese, Associate Professor
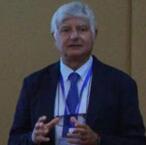
Department of Information Engineering and Computer Science, University of Trento, Italy
Biography: Maurizio Marchese is associate professor of Computer Science at the University of Trento in the Department of Information Engineering and Computer Science (DISI). He has worked in the field of web engineering and service-oriented architectures for over ten years. His main research interests are in social informatics: how information systems can realize social goals, apply social concepts, and become sources of information relevant for social sciences and for analysis of social phenomena. Lately, he has been involved in CIVIS, an EU project exploring the connection between social involvement in communities and responsible use of energy; and in ACANTO, an EU project developing a Cyber physical social network of older adults using robot friends.
Speech Title: Application of Clustering in Modeling User Preference for Leisure
Abstract: Multiple commercial systems focus on recommending suitable leisure activities to their users, yet little academic research has explored how to describe and model user preference for leisure. This paper investigates dimension-based and clustering-based approaches to modelling such preference. We rated a set of 135 leisure activities on 17 leisure dimensions in a user study and relied on these dimensions as feature vectors to cluster the 135 leisure activities using k-means and its fuzzy variant. Another user study collected user preference for the 135 activities to be used as the ground truth. The 17 dimension and resulting clusters were then tested as descriptors of user preference in a series of linear regressions. We expected that the user would tend to like or dislike all activities in a hard cluster, since such activities would be similar to each other, and the user would like them to a similar extent. We further expected that a partial membership in a fuzzy cluster would correlate with the user preference for an activity if the user liked or disliked the underlying cluster. The results suggested that clustering could indeed be used to describe leisure preference. Hard clustering appeared to be more effective than fuzzy clustering, but both of them could be combined for an even more effective approach to preference modeling than using them separately. Finally, clustering could be combined with the four most effective of the 17 leisure dimensions to account for 27% of variance in the user preference for leisure. Such result suggests that a combination of leisure dimensions and cluster membership can be used as leisure activity features, which may be suitable for and useful in content-based and hybrid leisure recommendation systems.